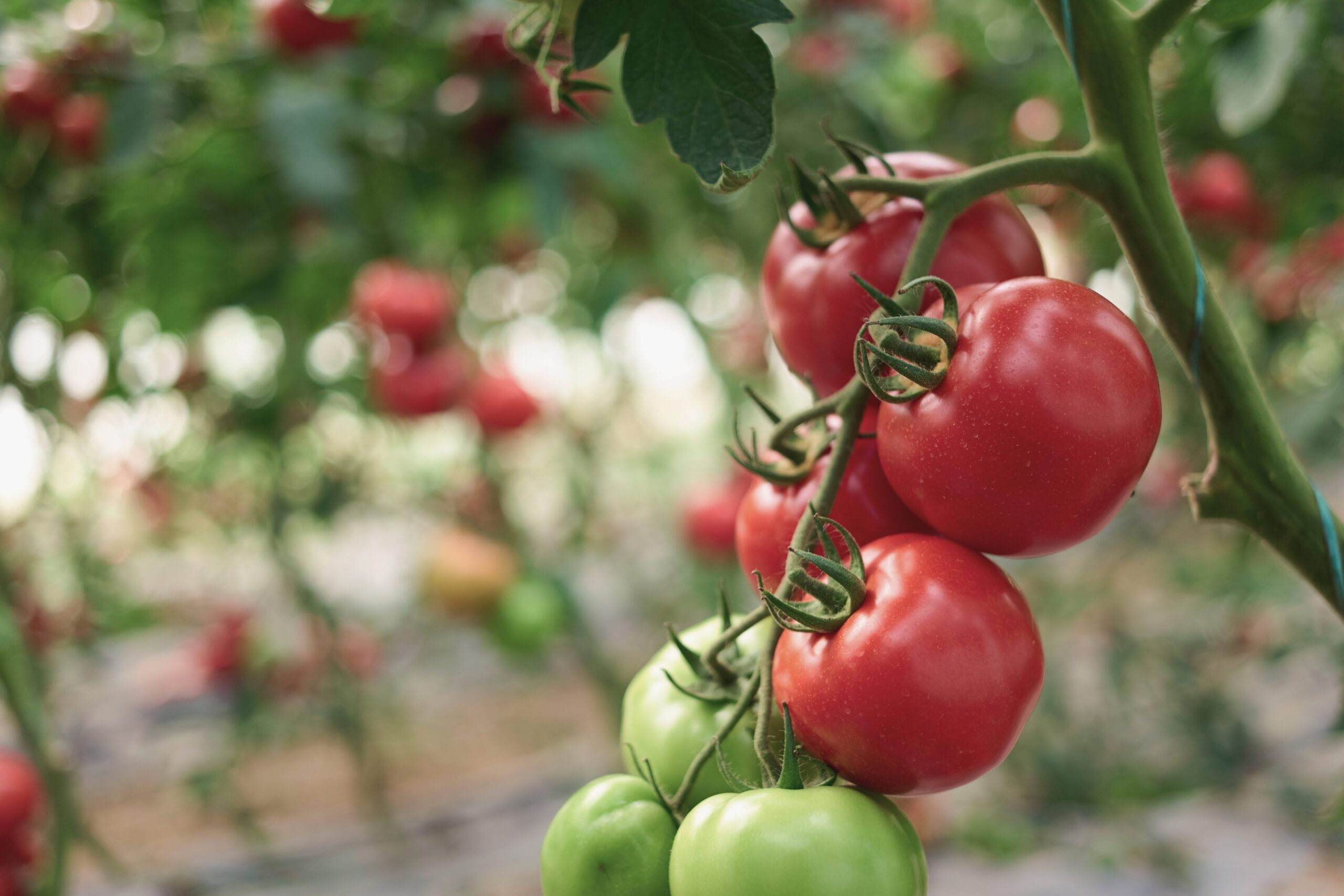
Operating fully automated packaging machines
Koppert Machines en Zonen B.V. designs and builds specialist machines for agriculture and horticulture, such as a fully automated packaging line for vine tomatoes with robots. Technolution Perform provided the integration of and the control system for this complex machine, which will realize a large reduction of labor costs and a greater weight precision compared to manual packaging.
Optimal distribution of vine tomatoes
The tomato packaging machine is equipped with industrial cameras to determine the size and thus the weight of the tomatoes. It has smart robot hands that can carefully pick up the tomato trusses. Conveyor belts carry the tomatoes past the cameras. The robot hands then fill the punnets. Every punnet must contain a kilogram of tomatoes, with a margin of at most five percent.
The packaging machine is fed by whole tomato trusses. The cameras scan the trusses and a 3D scanner registers the elevation profile. Software then determines the position of each truss, as well as the optimal cutting points, and estimates the weight and quality of every tomato. The tomato trusses are then conveyed through three robot stations, where they are cut and distributed across the punnets, so that each punnet contains a kilogram of tomatoes.
Technolution Perform developed all of the software and algorithms that operate the machine and the robots, and provided for the integration of software and hardware for Koppert.
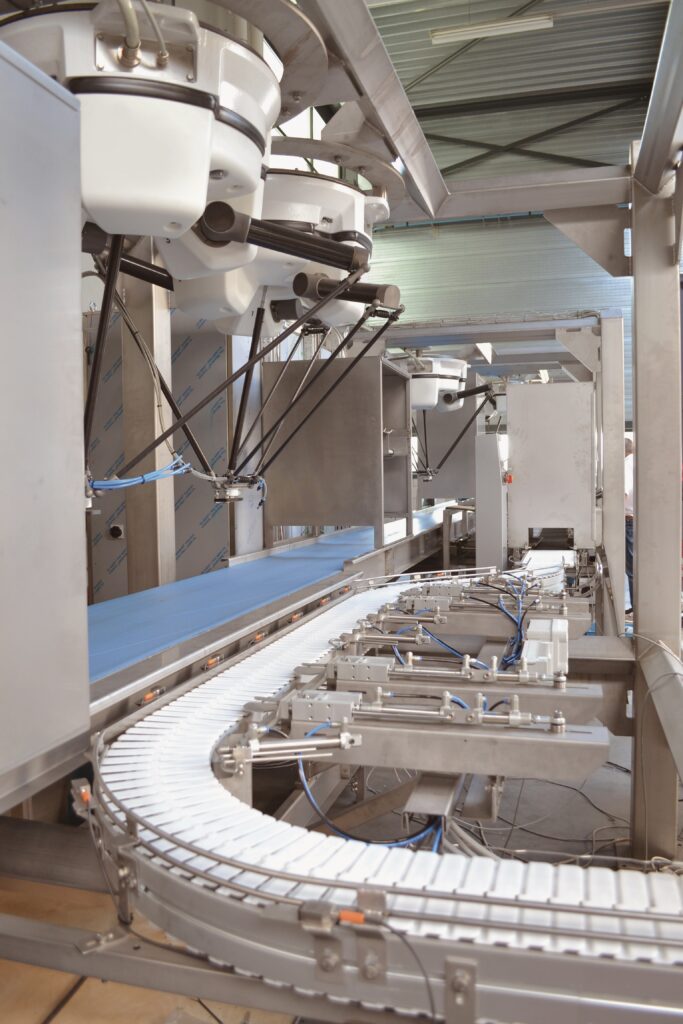
Artificial intelligence
Now that the machine is operational, we are researching the possible uses of artificial intelligence (AI) for the tomato packaging machine. Visual AI algorithms will be able to provide an even more accurate estimate of the weight and quality of the tomatoes. This will further optimize the efficiency of the machine.
Subsidied by
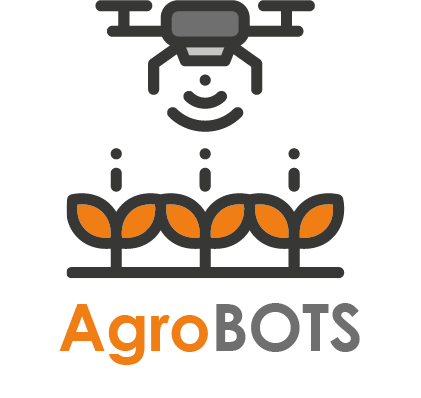
