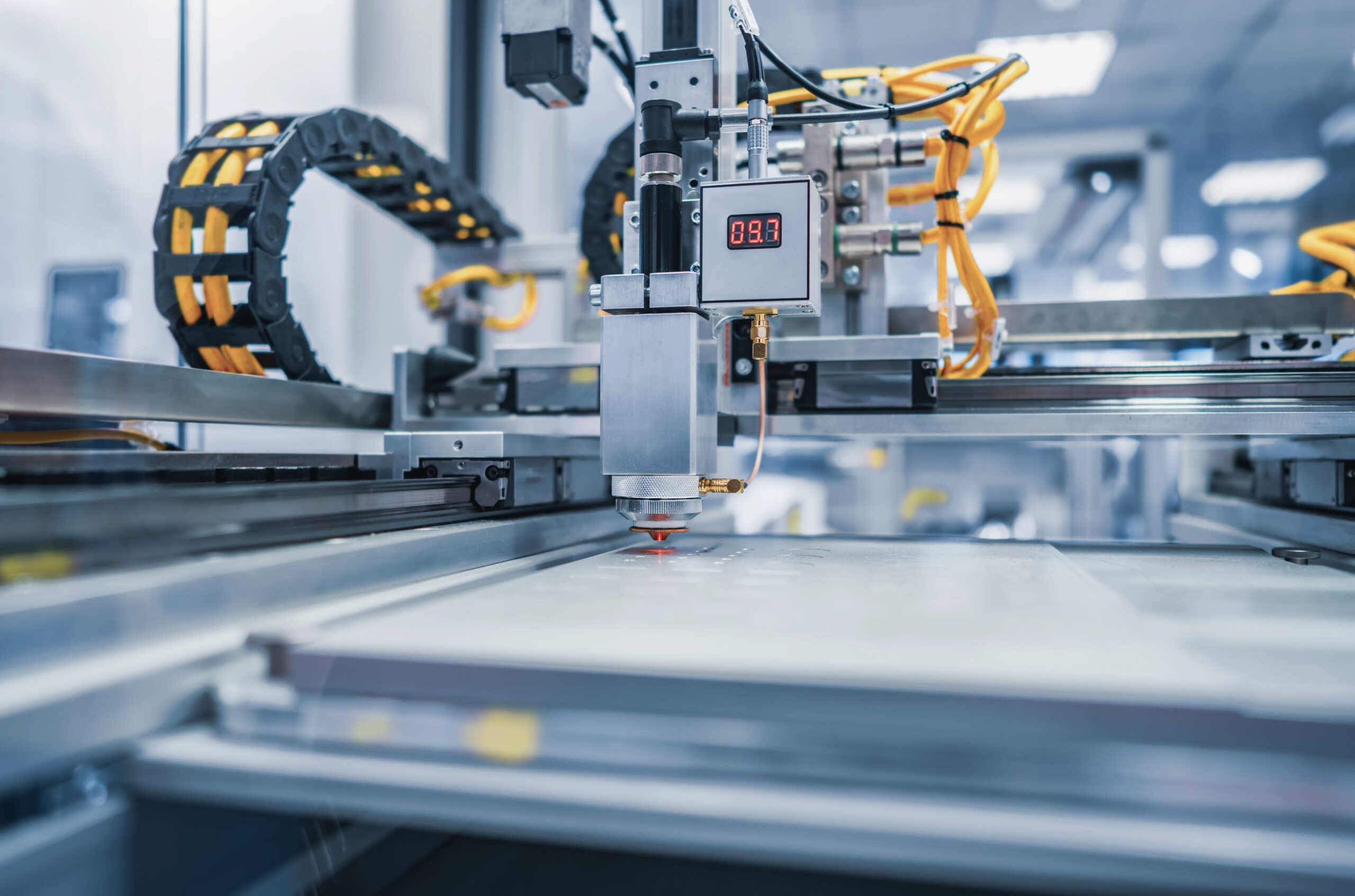
Custom-made technology for equipment manufacturers
Developing industrial equipment and machinery is an intensive process. Integration of new technologies is crucial for your clients, but it cannot put your time to market at risk. Technolution Perform can support you with high-end solutions for optimizing the efficiency, effectivity and continuity of your products. That means you can continue to focus on the total development of the machine, while the innovative technology ensures that your machines will meet your clients’ high expectations.
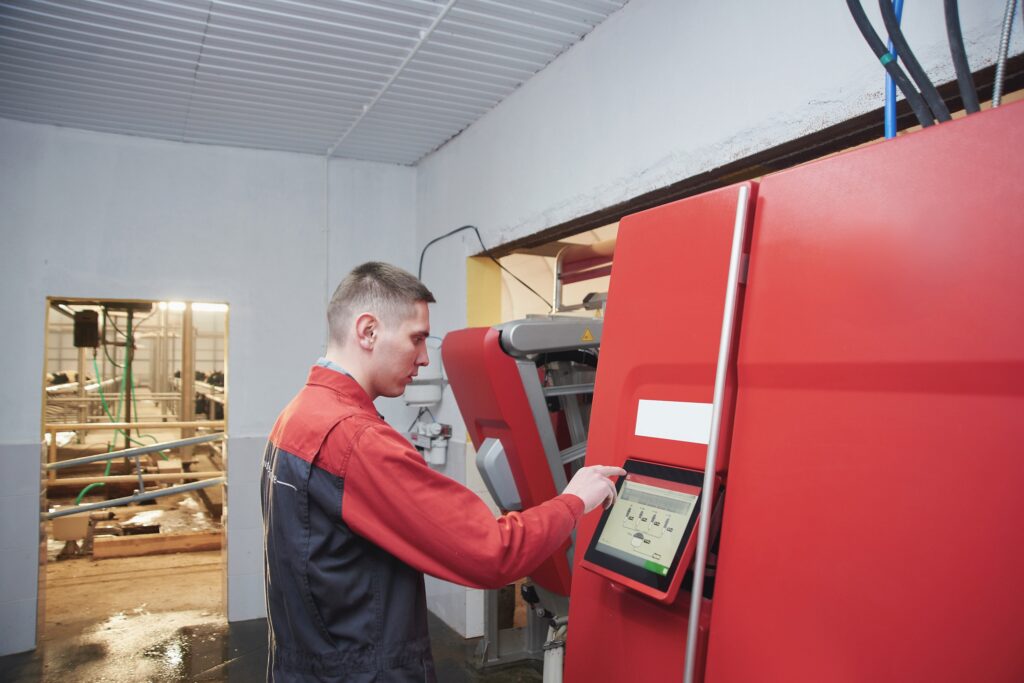
New and existing industrial equipment
New functionalities based on advanced technologies help you create added value for your clients. Artificial Intelligence for new visual functions, for instance. Or wireless communication through sensors and actuators, or online insight into productivity and state of maintenance. We develop high-end technology that enriches your products. Both for machines that you are developing from scratch, and for existing machines that you wish to retrofit.
Multidisciplinary customization
We work proactively with you on the basis of your requirements and wishes to make your product as distinctive as possible. We do this involving multiple disciplines, such as software, electronics, robotics and vision. Together with you, we look beyond the confines of our solutions to other disciplines in machine engineering and to the best way to adjust solutions accordingly. We are specialized in connecting Operational Technology and IT using edge computing, system integration and security. You can be sure of one thing: Technolution Perform will offer you a fitting solution, optimally adapted to your requirements and to those of your clients.